Efficient Development of High-Content Imaging Assays by Deep Learning
Genedata Biopharma Partner Symposium, Basel, Switzerland
March 7, 2024
Biopharma assay development aims to create robust assays that can run consistently over weeks on automation platforms, and are monitored by well-defined quality control metrics. Usually, such assay development is an iterative process, requiring multiple optimization rounds to determine ideal assay conditions. The development of high content imaging assays typically involves in addition an iterative development of image analysis due to their complex read-out. We report here on an analysis workflow that eliminates this bottleneck.
In a speck formation assay, the best combination of assay window, assay sensitivity, robustness and reproducibility was sought by varying incubation times and the dosing of both inducer and stimulus. For image analysis, a traditional image processing workflow and a deep learning-based workflow were compared. We describe the concepts of both workflows, and assess the benefits of switching from the traditional image analysis workflow to an integrated workflow using a deep learning classifier to quantify the relevant cellular phenotypes. In particular, we highlight the robustness, efficacy and flexibility of the deep learning-based workflow, and its selection as the primary analytical method for this speck formation assay and others at Roche.
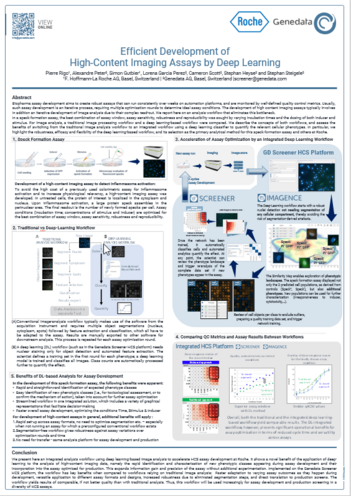